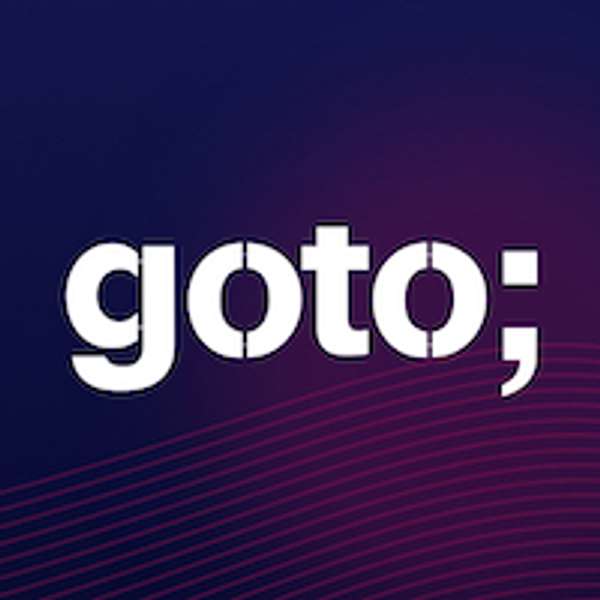
GOTO - The Brightest Minds in Tech
The GOTO podcast seeks out the brightest and boldest ideas from language creators and the world's leading experts in software development in the form of interviews and conference talks. Tune in to get the inspiration you need to bring in new technologies or gain extra evidence to support your software development plan.
GOTO - The Brightest Minds in Tech
Your AI Survival Guide • Sol Rashidi & Joe Reis
This interview was recorded for the GOTO Book Club.
http://gotopia.tech/bookclub
Read the full transcription of the interview here
Sol Rashidi - Author of "Your AI Survival Guide", Keynote Speaker & "Forbes AI Maverick & Visionary of the 21st Century"
Joe Reis - Co-Author of "Fundamentals of Data Engineering", CEO at Ternary Data, Keynote Speaker, Professor & Podcaster
RESOURCES
Sol
https://twitter.com/Sol_Rashidi
https://www.linkedin.com/in/sol-rashidi-a672291
https://www.solrashidi.com
https://solrashidi.substack.com
Joe
https://www.linkedin.com/in/josephreis
https://github.com/JoeReis
https://joereis.substack.com
http://josephreis.com
Links
https://learning.oreilly.com/get-learning/?code=LEARNGIT23
Trisha & Helen: https://youtu.be/pfinplXtrkA
DESCRIPTION
Join Sol Rashidi, an AI deployment pioneer, offers practical wisdom on navigating AI's challenges in a conversation with Joe Reis. Her new book, "Your AI Survival Guide," provides actionable insights for individuals and businesses venturing into AI integration, emphasizing hands-on learning and a measured approach to address cost and sustainability concerns.
RECOMMENDED BOOKS
Sol Rashidi • Your AI Survival Guide
David Foster • Generative Deep Learning
Phil Winder • Reinforcement Learning
Mustafa Suleyman • The Coming Wave
Ashley Peacock • Creating Software with Modern Diagramming Techniques
Bluesky
Twitter
Instagram
LinkedIn
Facebook
CHANNEL MEMBERSHIP BONUS
Join this channel to get early access to videos & other perks:
https://www.youtube.com/channel/UCs_tLP3AiwYKwdUHpltJPuA/join
Looking for a unique learning experience?
Attend the next GOTO conference near you! Get your ticket: gotopia.tech
SUBSCRIBE TO OUR YOUTUBE CHANNEL - new videos posted daily!
Intro
Joe Reis: Sol Rashidi, how's it going?
Sol Rashidi: It's going well. Thanks, Joe Reis.
Joe Reis: Anytime.
Sol Rashidi: How are you?
Joe Reis: Doing good. Just did a quick workout and walked my dog. And here we are. So, how are you doing?
Sol Rashidi: I'm good. I did the same. I did my sprints this morning. I didn't lift this morning. But the sprints kind of knocked me out of my fog a little bit. So that's always good news. That feels good.
The Evolution of AI: Democratization and Domain Specificity
Joe Reis: Good news. Awesome. Well, we have a lot to talk about. So let's get on to it. First off, for people who don't know who you are, do you want to give a quick intro?
Sol Rashidi: Sure. I say I'm a recovering slash/retired C-suite executive. I was a Chief Data and AI Officer back in 2016 for Royal Caribbean. Then the Executive Vice President and Chief Data Officer for Sony Music. And then the Chief Data and Analytics Officer for Merck Pharmaceuticals. And then the Senior Vice President and Chief Analytics Officer for Estee Lauder. But as of eight months ago, I no longer work for enterprise. So, it's been a new adventure. I've been doing new things these past eight months. But I have been in the data space since the late 90s. I've been doing AI, as everyone puts it in quotes since actually 2011. I helped IBM launch Watson. I've been involved with design, conceptualization, development, and pushing POCs into production for quite a few years before everyone caught on board. So it's great to see that our fields and our spaces are still loved. And it's getting some hype, of course. But it's out in the open. I think there's been a massive resurrection. So, I'm enjoying it very much.
Joe Reis: That's good. So, you've been in the data and AI space for a long time. We'll get into your book in a second here. But walk me through some of the early days and kind of how we got to today with "AI?" I think you have a good perspective on things and real-world deployment. So, what have you seen since you got into it?
Sol Rashidi: So it's interesting, I think that there's a great divide between the haves and the have-nots. When Watson released Engagement Advisor, Watson ENGAGEMENT ADVISOR, it was no different than ChatGPT. It was a natural language processing application layer, with a user interface that was really easy to interact with, sitting on top of a big data ecosystem. Back in 2011, remember, like, compute, storage, those things weren't nearly to the degrees they are now. But the biggest differentiator between 2011 and now was, that if you wanted to leverage any of the AI capabilities, it was predominately B2B. It was only available to other companies and other enterprises. And so there was a lot of embedded AI back in the day. There were a lot of large companies who could afford the POCs, like the $2 million sticker prices on a POC. However, the application, the technology, and the advent had not been made available or democratized to direct consumers or citizens.
So fast forward to where we are now, what I really love about the space is you no longer have to be an enterprise to enable it, adopt it, or release it. I use five to seven different tools on a daily basis to give me time, capacity, bandwidth, and productivity back. Not to replace what I do, but just to accelerate what I do. I always say it's easier being an editor than an author. So I'm just glad that these capabilities are available to everyone, and it's no longer just enterprise. So I would say that's the first major leap. I think, too, with large language models. I've always said they're great, but they're large, and the general context isn't perfect. I'm very excited about sort of a new space that's created, which is very domain-specific. So commercial banking is very different than the payer-provider or patient network, which is very different than the Mahr tech and digital campaign domain, which is very different from than supply chain or manufacturing. And so, I'm super excited to sort of see the next leap, which is domain-specific LLMs. Or I think you'd even mentioned they were called small LLMs, which I don't know if I'm a big fan of that name. But I think these domains…
Joe Reis: Small language models.
Sol Rashidi: I don't know if small language models really convey what domain specificity is going to do for us, but like, that's what I'm excited about next. Just to be able to, again, accelerate and have to bypass all the training, tuning, and all that other fun stuff. I would say the biggest thing that excites me about where we were and where we are now, is it's no longer just restricted to enterprise, [crosstalk 00:04:33].
Why Write a "Survival Guide"?
Joe Reis: Right. It definitely seems like the tools are readily available for everybody. Yeah, and I guess we'll get into your book now. It's coming out imminently. It's called, "Your AI Survival Guide: Scraped Knees, Bruise Elbows, and Lessons Learned from Real World AI Deployments." So, why the heck write a book on this? Why not write about why AI is awesome, and it's really easy to do, and all that fun stuff?
Sol Rashidi: The playbook, the guide. I think there are plenty of people talking about... I think there are two sides to camps. But there's plenty of people talking about how amazing AI is. And then I think there's those talking about how it's the end of the world. Regardless of what camp you're in, unless you're a researcher, or academic, you know, you're paying attention to the news of how amazing it is and how it's going to change the world. But very few people have actually gone through the failures, the scraped knees, the bruised elbows of what it's like to actually push something from POC to production. Because either things don't launch, or they're in perpetual POC purgatory. Those are things that I've learned along the way, having created these applications, and pushed them into production. And a lot of them are still alive now. I know a few have been retired, just because they lost the subject matter expertise to actually maintain and support the applications that we built.
But for the most part, a lot of them are still operational and alive. When you go through that process, it's amazing how you could read something about a strategy or you could read something about a framework. However, the actual deployment and application is a completely different business. I think that's where cultural norms, cultural hesitancy, the people aspect of it, it's never the technology, you can solve those problems. It's always the people. And especially when you're in a large environment, and you have to work with folks from other cross-functional teams, but you've got the naysayer, or the curmudgeon, or the person who's overly optimistic, but has no idea what they're doing. Those are the things that frustrate you and become the roadblocks. So, I wrote a book on the realities of it. And not because I'm some expert, or... I think if anyone says they're an expert, you should be aware and be careful. But I've gone through the failures, I've gone through the faceplants.
The book is really around, it is amazing, here's how you get started. But here are things I learned along the way. Here are the mistakes I've made. Here's what I faced. If you're in this environment, you have to do it this way. If you're in this environment, you have to try this situation. The goal is to help anyone who's even remotely interested in starting, who isn't technologist... I think that's key. How do you even start? And what do you watch out for? How do you get your team and your leaders to evangelize? So you could be a person who owns a $150 million stainless steel manufacturing plant in the middle of Minnesota, and you're like, "Well, I want to use AI to automate and help my sales team." Or you could be a $2 billion company that just got bought by private equity with EBITDA margin pressures. Right? How do I become more efficient? Or you could be a large enterprise. Like, how do you even approach strategy?
Because I've had to go into enterprises. And I'm like, okay, you have all these use cases, and they're struggling, they don't know how to convert it over into something actionable. And, like, well, seven of your nine use cases, you can't do. And they're like, why? I'm like, you don't have the maturity or the readiness. Your infrastructures like this. Your data is like this. You don't have the talent. So you can do the POC, but if you want to push anything into production, seven of these nine are not going to do it for you. And so the book was really more of a realistic guide for just like how it's done.
Narrowing Down AI Workloads: Practical Strategies for Companies to Avoid POC Purgatory
Joe Reis: That's really interesting, because I think, right now, we're sort of at another hype cycle with AI. I don't think it's debatable. But it seems like every company wants to get into AI, whatever that means. How would you advise companies to choose a workload, or, I guess, an area to focus on, where they can avoid being stuck in, I guess what you called POC purgatory?
Sol Rashidi: I think one, there is an element of risk exposure to the type of strategy you choose. And then three, making sure whatever you choose is internally focused and not externally focused. So, let's just start with strategy. There's a growth-based strategy for AI, an efficiency-based strategy, an efficacy-based strategy, a knowledge-based strategy, and an efficiency-based strategy. Each of those strategies you choose is based on the reality and the maturity of your current organization. And part of that is, do you even have your data aggregated? Forget hygiene, forget cleanliness. Like, is it even a single location for you to pick up and start training? Or is that even an exercise you have to go through? Or from an infrastructure perspective, do you have one dude that you ask all your IT questions to? Or do you actually have a team of three to four individuals with, like, true developers and engineers, and QA folks that you can go to?
All strategies are dependent on your existing maturity. Because I stepped into the mud of picking a strategy and a use case, and we didn't have the organizational maturity for it. So I had to put 14 months of effort into building a data ecosystem, so I could even launch the use case. Like, I'm never going to do that again. So I'm always about, being realistic about what strategy to pursue. And then the second is around risk tolerance and exposure. You know, if you're in a conservative environment that's not really risk-taking, you're not going to pick an externally based use case. Like, you're not going to go to market. You haven't developed the muscles yet. Pick an internally based use case. Because you could screw up with your folks, you can't screw up with clients and customers.
Then the third is, and this sounds so common sense, but common sense isn't really commonly applied. Pick an area that has fundamental pain points and fatigue. I can't count how many times I hear, all right, well, our CRM system is crap, there's not enough data in there. I don't know if I should continue to invest in my sales org, or if I should reallocate my capital and invest in my equipment. But I do know that sales will eventually lead to business, growth, and margin, but I gotta be able to grow, so I gotta invest in the equipment. But the data we have in the CRM system is crap. Our salespeople are not as efficient. I need to hire a lot more to be able to meet the capacity of what's coming on board. I'm not sure what decision to make.
All right, well, applying your capital towards equipment, if you're already growing to a certain degree, sounds great, because you can build efficiency, and you can build productivity, capacity, and bandwidth within your sales organization. But what are they struggling with? And if you pinpoint that the struggle is, CRM's data is crap, we have no idea where lead gens are in the pipeline and the funnel, because they're not entering in the information. Well, to me, that's an easy use case. It's totally solvable. It requires processes. There are off-the-shelf solutions that can help you with that. That's probably a place to start before you take on this big magnanimous thing. So I'm always like, think big, but start really small, exercise those muscles. Let people get to know that it's doable. It's not taking over the world. Let them believe in it. Then you start expanding and scaling it.
So if you consider those three things, like, you're at a solid start for people who haven't started yet. And I don't think that there's any element of FOMO that should kick in. Do something about it, but don't rush into it. Like, I hear some of the management consulting firms, like, if you don't do AI, you're gonna die. Easy. Like, that's not the case. This isn't going away. Yes, there's a lot of hype and marketing around it, because every company is putting a logo on it. But if you take that aside, like, this is just another evolution. We went from on-prem to cloud. It took us five, or six years before we crossed that chasm. And here's the fun fact, 85% of most IT infrastructure costs are still on-prem. We just assume everyone's on the cloud. And it's not, it's only 15% of the market share. We've been doing cloud since what, the mid-2000s? So I think AI is going to be something similar, where there's going to be a ton of traction. But there's still only a small handful of enterprises that are leveraging it. And you get a lot of startups that are building businesses out of it. You've got the rest of, you know...the slew in between, they're still kicking the tires and figuring out where to apply it. So be deliberate, be thoughtful, and get your team on board. Think big, but start small.
Deciphering Legitimate AI Solutions: Insights from Sol Rashidi's Vetting Process
Joe Reis: The FOMO is a real thing, though. It feels like every company is getting...seemingly every company, is getting pressure from its board of directors or its CEO to become AI capable, to do AI. I'm doing this in air quotes right now. And you point out too, that it feels like every tech, data, and software vendor's now suddenly slapped AI onto their products, right? They probably have an AI domain address, where they maybe have the little thing with the stars all over it. It's the magic fairy dust that indicates AI. But if you're a company that has these directives to start doing AI, and probably quickly, and there's no shortage of vendors claiming to do AI. How do you suss out which vendors are, I guess, legit, and what vendors are probably not so legit in the AI space?
Sol Rashidi: My first rule of thumb before I buy any vendor, like, any software from any vendor, is I never talk to sales and marketing. I’m like: Bring in the solution architects, bring in the engineers. I want to understand which feature and functionality are we tagging as AI and why. Because my running joke is, do you just have a bunch of SQL scripts that are running business rules that have been established, that although profound and large, they're still SQL scripts running business rules? Or is there embedded intelligence in this? And if so, where? So I'm probably the worst person to talk to because, like, vendors love and hate me because once I'm an advocate, I have no issues mentioning their name. However, the vetting process to go through my BS detector is quite extensive. And I don't talk to the sales team. I talk only to solution architects and engineers because they cannot lie about what is and what is not. So they're the ones that I talk to.
Joe Reis: Interesting.
Sol Rashidi: I believe in the five whys. I don't solve for symptoms, I try and find the root cause. And so I don't take anything at face value. Sometimes it pisses my former teams off. Because I'm like, okay, well, tell me about this. Well, tell me about this. Sometimes you can feel like I'm not trusting them. But that's not the case at all. I just have a very scientific mindset, and I go into forensics. I just ask questions because I want to legitimately understand it. But in that process of asking questions, there's some reason for the magic number five. I can either figure out if there's something solid here, or if the explanation, the argument is starting to disintegrate. And then that's when I knew to go a little bit deeper. And inevitably, at four or five, I always get the, well, I'm not sure. I don't know. I'm like, okay. That's when I know that I'm not talking to the right individual. And they're using marketing terms on me.
So, I typically bypass that and I say, just bring the solution architects and engineers in. I want to have a discussion. And I just keep going and going and going. And by the fifth question, I have a good radar of whether or not it truly is or is not. So if you're not a technologist, and I would never consider myself with the likes of like, you Joe, or some of your peers, I'm sort of in between. I'm not totally business-minded, I'm functional. I wrote code, I haven't written code in, like, 20-plus years. I was told I was never allowed to touch code. But I love the space. I love managing teams. I love architecture. And just building things. And so I've always been in that weird role in between. But I can go toe to toe with an architect, and I can go toe to toe with an engineer and suss it out. So those who are functional and non-technologists, I would say at face value, don't believe anything's AI. And I would say, great, have a handoff with the marketing team, or the sales team, and say, I want to talk to the solution architect and engineer. And then bring someone from your team to help you suss out whether it is or isn't.
Open Source vs Managed Services in AI
Joe Reis: Sure. And then what are your thoughts concerning choosing open source, versus either third-party or managed services?
Sol Rashidi: I am a big fan of open source. However, in the positions that I've held, I was never able to leverage open source and push anything into production. So I think open source is great for individuals, small companies, to leverage, and accelerate, and create, and build, and innovate. Unfortunately, within the enterprise, I've never been able to leverage anything open source. Because there's this stigma around instability, vulnerability, and increased service attacks. And so everything I've done in enterprise had to be closed source. Sometimes we'll leverage and borrow something from open source to be able to create a functional prototype or a POC quickly and say it works. Then I'd have to find a comparable closed-source mechanism and push that into production.
And because enterprises don't necessarily have all the right talent in the right place, to do the right things, managed services are 1000% needed. I don't think I've ever crossed an enterprise... And even my fellow peers, whether you're chief data, chief analytics, chief data analytics, or chief AI, whatever officer you are, CXO. If you're an enterprise, you leverage managed services. Because handling that end-to-end stream is extremely difficult.The top talent are the individuals that know how to do their craft or their component well. They're not joining an insurance company. They're not joining a commercial banking company, per se. And so that's where managed services come in.
Joe Reis: Interesting. I think it's one of the dilemmas too, right now, especially... You know, what seems to be separating this latest crop of AI products versus the previous generations of AI tools and hype cycles, is just the cost of training these models is substantially higher than it used to be. Do you recommend that companies train their own AI models, say, a large language model? Or would you leverage a third party for that?
Sol Rashidi: I would leverage a third party. That's my preference. There are sort of three layers in the cake. You've got your bottom layer, which is mostly focused on computing, generating inference, and going through all the software components to develop your own foundational model. You can do it in any number of cloud providers. You really have to know what you're doing to be able to manage not only every single component but develop your own foundational model. And in the enterprise, I haven't found a specific need where you needed to do something like that from scratch. I think then there's that middle layer, where it's managed services, where you leverage a preexisting foundation a model. Then you go through and you feed it your own personalized data. And then you put in components around cloud security and cloud ops. And then there's an embedded-gen AI application. Of course, there's training, and fine-tuning, and inference, all that other jazz. That seems to be the sweet spot for most corporations and enterprises, even medium-sized companies.
But I'm a big fan of leveraging what's already there. So if there's already a product, if there's already a capability... And I don't want to vendor drop any of the names, but if there is a preexisting off-the-shelf application that could...and I don't wanna say plug and play because it's never that easy. I always say, if other giants have solved the problems for you, why are you trying to accomplish the goal that someone else already has? You know, we read a ton of leadership books, you're an avid reader. And part of that is, is you're learning from others who've gone through the process, and see how it applies to you. And I think that's very much alive and well right now. If others have already invented it, the novelty comes from being able to what I call Frankenstein stitch together different components that have already been solved for that novel solution. I think that's where the magic happens.
The Next Chapters of AI
Joe Reis: I'm curious to see what kind of happens over the next couple of years in terms of the adoption of AI. I think, last year, it was all about sort of the crescendo of the AI. And now I think we're starting to get to the brass tacks of actually deploying this stuff. What do you think is gonna happen over the next couple of years?
Sol Rashidi: I think there's sort of three legs in that stool. I think, no one is looking at the cost of running the workloads. I was recently at a client site where they brought me on board to assess because they had applied an AI application. They generated around $600,000 in revenue. And so they were happy with the results. And I said, "Okay, well, what was your cost to run the workload?" And they're like, "What do you mean?" I'm like, "Well, what was your cost?" They couldn't answer the question. And so they were using one of the big vendors, right? So if you take a look at Google, Amazon, Microsoft, let's say one of those three. And I'm like, "Wait, so you guys did not calculate the cost to run the workload that helped you generate 600,000?" And so they had to go through this painstaking process of stitching together, I think it was like over 100 different skews, only to find out that they'd spent $3 million to make $600,000.
Joe Reis: Good job.
Sol Rashidi: Where's the ROI on this? But they weren't looking at it. And no one was highlighting it. And I'm like, then how are you measuring whether or not this is a good investment? So I think in terms of the first observation, no one's being cost-conscious yet, but we're eventually going to get there. I think they're going to understand that the cloud costs have exponentially gone up, not incrementally gone up, with the AI applications that they're trying to introduce, train, and deploy. So I think people are gonna get more cost-conscious, which is just going to put more pressure on all the chip providers to be a lot more efficient, and infrastructure providers to be a lot more scalable, agile, but in an efficient manner. And it's just not there yet. So cost consciousness.
I think the second is, that there's going to be a rude awakening for anyone who has sustainability and an ESG initiative, with being an AI-centric company. Because the two right now are in direct conflict. Because of the amount of energy and resources that it takes... It's crazy, there are some qualitative, I don't know if they're quantitative facts that are sprinkling around. But to run a single prompt mid-journey is like leaving your office lights on overnight. Or every prompt entered is, like, throwing a plastic bottle into the ocean. If the entire United States were to turn into an electrical grid, we still wouldn't have enough power to power Fortune 1000s If they were all running AI applications. Like, the tremendous energy resources it's going to take are in direct conflict with all the sustainability and ESG initiatives that are out there. And no one's talking about that yet. So that's something that I think in the next year or two, will start bubbling to the surface.
Then third, I think right now, you know, the LLMs are the prom kings. I'm curious to see who's going to be the prom queen, and who is going to outshine the prom king. I don't think it's going to be the LLMs anymore. I think it will be that middle layer of managed services, soup to nuts, or a lot of the applications that are going to be pre-built, that people are going to leverage, so they don't have to train or build their foundational models and leverage LLMs from scratch. Those would be the three that I would say are up and coming. But we'll see. Who the heck knows?
Joe Reis: We'll see. I know Google, at their latest conference, they are pushing agents hard, and so forth. So, we'll see. It's anyone's guess.
Sol Rashidi: I mean, even with Amazon and Q, right, or with Rufus, it's like, everyone's pushing agents. Because that hurdle, that obstacle of getting alignment, agreeing on a use case, finding the right talent, ring-fencing budget, understanding, like, what it is, the problem that you're going to solve, getting the business involved because you're still human in the loop and you need those knowledge workers, you need their experience, to do the development, to build it to a point where the threshold meets your minimum of viable acceptability criteria. And that's just to get the PFC going. Like, pushing it to production is a different beast. So that in and of itself, in a small environment, should take a few months. It can take up to a year in a large environment, in an enterprise. So I think if there's any way of short-circuiting that, it would be beneficial for everyone.
Empowering Teams for AI Integration: Insights on Skill Development
Joe Reis: Obviously, this is a podcast, it's geared more toward developers, and so forth. And kind of anecdotally, the other day, I was talking to a friend of mine, and he was saying that he was just at, like, a big JavaScript conference, a lot of web devs. And there was a big interest in these devs, of learning AI, and I think leveling up the skill set there. I think there's also FOMO maybe in parts of the developer crowd. And you've led very big teams. What's the biggest team you ever led?
Sol Rashidi: Eight hundred and thirty-two.
Joe Reis: Okay. That's a small town.
Sol Rashidi: Wyoming.
Joe Reis: Probably where I'm from, you're the mayor. You've worked with developers and technicians throughout your career. Let me give you this scenario here. You're running maybe a dev team that may have some core technical competencies, maybe some data competencies, but maybe not AI competencies. How do you level up your team? How do you suggest that they also work to level up their own skill sets, to, I guess, be able to work with AI?
Sol Rashidi: Honestly, there's no better teacher than experience and failing. I do think success is a S-H-I-T-T-Y instructor. Forget, like, how I've upskilled. But if you're an individual, whether you're working for a corporation or not, you could be with a startup, you could be your entrepreneur, or you could be starting a business that's AI-based, but don't know where to start. I think the easiest and best way is to understand your own life, where you're struggling, where you need time back, where existing capabilities exist, and start building solutions for your own life, leveraging AI.
I'd mentioned earlier that I use, five to seven different tools. And there's auto-scraping stuff that I use. There are auto responses that I use. There's even summarization, like, Chrome extensions. Like, I read every morning for like an hour and a half. And I don't have time to read through all the white papers, articles, research papers, and everything that's coming out on the web. And so I had the summarizer that within milliseconds, summarizes a web page, or a white paper, or an article. I read the summary to see if it was even worth my 25 minutes of investing in this nine-page white paper. So I would say, personally, find areas in your own life that could leverage and could use some help, and then find ways of implementing it. Whether you've leveraged an API, and you build the application yourself, or whether you take something that's off the shelf, and you embed it within your life. Be sort of your guinea pig. And that will help you understand how things and mechanisms are working, that are already available out there.
Then I would even go in as a second layer, to understand how they were developed. What's embedded behind the scene? What's the code source? Because you can easily get the code source, right, with a few prompts. And start understanding how they've structured embedded intelligence within the AI application that you've now embedded in your own life. So be your guinea pig and be your teacher. Once you start understanding the different applications, it's funny how the light bulb goes on. You can immediately spot a problem and go, "Well, I can either leverage AI for it, or, no, I'm going to use RPA for this one. That's easy.
You can spot opportunities, and then when you spot opportunities, you start researching the players who play in that space. And then you can start the journey of experimenting. But you can read all you want, you can study all you want. It's still theory. It's still practice. It's like going to school and learning algebra. Unless you have a real-world application, like, no one remembers multivariable calculus. We don't apply it today. So for me, I think the academics are great. Take a few of the free courses, tons are available, there's no shortage of links on LinkedIn. Or if you need suggestions, ping me, I'll give them to you. But don't spend too much time just taking available courses. Apply it to your personal life, and then introduce it slowly into the company life that you live in. I would say that's the best teacher. It may sound hokey, but it works.
Outro
Joe Reis: That's awesome advice. Well, thanks, Sol. It's been great chatting with you. Congrats on the book, by the way. I know how hard it is to write one. It's a lot of work. For people who want to learn more about you and your book, how can they do that?
Sol Rashidi: There are three ways. So the book is available on Amazon right now. Kindle is available. The book will be released on April 30th. LinkedIn, I've recently become very, very active. So you can always follow there. Thanks to Joe's advice, I now have a newsletter. And that's on Substack. So it's solrashidi.substack.com. So, the book is on Amazon, and I'm on LinkedIn and Substack.
Joe Reis: That's cool. And a lot of people seem to like your content too, by the way. I think you get a lot of great engagement and great discussion. So that's awesome.
Sol Rashidi: It's a new world for me. I'm used to doing the work, not writing about the work. So even the process of writing the book, of taking everything that's in my head and putting it on paper, was crazy. But now even writing the content, I'm blessed and fortunate. I guess, yeah, you're right, people are liking it. I never thought they would. It's a little embarrassing for me to write. I know you're a pro at it. I'm still very bashful when I write. I'm like, "No one's gonna care about this."
Joe Reis: You are a pro now. People are paying for your work. So, welcome to the club.
Sol Rashidi: Bow down to the leaders.
Joe Reis: Awesome. Well, it's great chatting with you. So, take care.
Sol Rashidi: Thank you. I appreciate it, Joe Reis. Thank you so much.